Preparing for AI
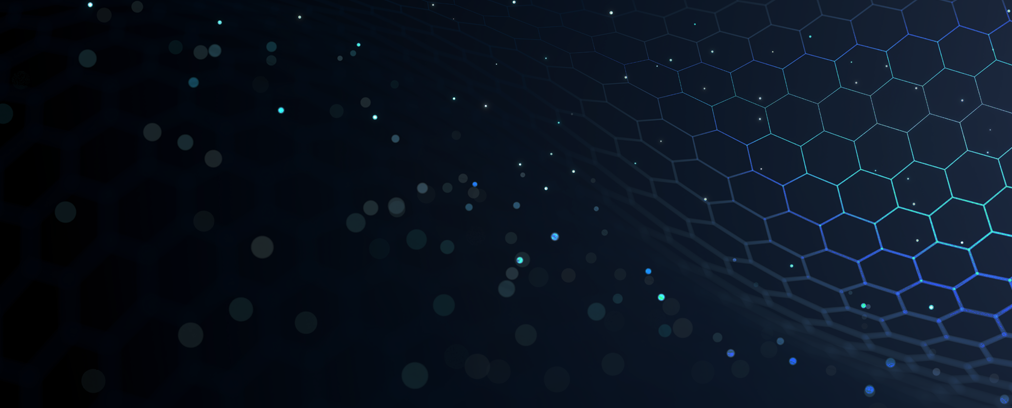
Implementing AI for the Business
While AI-enabled businesses have flourished for the last decade, recent attention from the popularity of Chat-GPT and other AI tools has energized the conversation about the role of AI in business and consumer interaction. However, what appears to be a sudden emergence is the cumulative work of nearly 75 years, and a rapid expansion over the past 20. The growth in accessible large data volumes, processing power, and complex algorithms have worked together to turn theory into practice. Add to these the recent work in deep learning neural networks and natural language processing (NLP), and AI has become a realistic and reusable toolset across different business domains and dimensions. AI has become an essential part of modern business strategies, and organizations that leverage AI effectively can achieve significant competitive advantages. So how can businesses prepare for and implement AI to gain the most immediately and long term?
Brands desiring to gain full and significant impact must prepare methodologically with an AI strategy that drives KPIs at scale. Brands must:
- Assess current state of the business and AI intersections
- Assess and prep data for use
- Invest in AI infrastructure and technology
- Upskill and staff for AI practices
- Pilot, evaluate, and scale
Artificial intelligence (AI) has become a transformative force in the business world. It has the potential to revolutionize the way businesses operate, enabling them to be more efficient, effective, and innovative. However, adopting AI is not a one-size-fits-all approach. The steps a business must take to leverage AI in future performance will depend on various factors, such as the nature of the business, the industry it operates in, and its existing tech infrastructure.
The Cost of Inaction
The scale and complexity of preparation drives brands to either postpone dipping into AI practices, or to create small disconnected pilots that never scale to measurable impact. However, the cost of not implementing AI for a business can be significant.
Missed Opportunities for Growth and Innovation
AI-driven analytics tools process and analyze vast amounts of data, helping uncover hidden patterns, trends, or customer preferences. Sentiment analysis tools can gauge customer emotion and reactions leading to new campaigns or even product lines. AI-driven recommendation engines can suggest relevant products that drive sales. Chatbots can offer personalized support, answer frequently asked questions, and even guide customers though purchasing processes. All of these opportunities to tailor offerings to specific segments are driven by algorithms processing customer data volume beyond human analytics. These unaddressed opportunities equal lower engagement and sales than necessary, and ultimately, lower market share.
Increased Operational Costs
AI can identify and remove inefficiencies, errors, and even manual processes that increase costs when unaddressed. Manufacturers can use AI to control for defect rates, rework, and waste. For example, businesses with significant fraud risk may be able to better detect and reduce loss with AI fraud detection. Without these enhanced processes, the business continues to carry unnecessary expense.
Inability to Compete with AI-enabled Competitors
While missed opportunities for growth and efficiencies carry measurable costs, businesses will likely face increased competition from competitors who leverage AI to improve operations and customer experiences. With ongoing pursuit of use cases and experimentation, competitors will be faster to explore new marketing channels and techniques.
Balancing Efforts Across AI Dimensions
To balance short- and long-term AI planning, businesses must take a strategic approach that focuses on both. This involves developing an AI roadmap that outlines specific AI initiatives for the immediate tactics and strategic goals. For example, a business might prioritize immediate needs by implementing AI-driven chatbots to improve customer service, while also investing in data scientists and assessing data quality in key dimensions (e.g., CDP, CRM, Google Analytics) for large-scale AI.
If implementing a custom NLP solution to automate customer service is beyond the immediate reach of many brands, there are solutions that can drive performance in this area without bringing on a full team of expertise and tech infrastructure–with limitations.
Integration Strategies
Full Business Alignment
The first step a business must take to leverage AI in future performance is to develop an AI strategy. Brands review business goals, strategies, and tactics in order to determine ways in which AI can help achieve them. In addition to overall industry strategy alignment, the AI strategy must address the strengths, weaknesses, and unique context of the organization.
Greatest impact requires identifying areas where AI can provide the most value, prioritizing use cases, and defining success metrics that align with the organization’s vision, mission, and goals to drive sustainable growth.
Integration into Existing Workflows
Long-term strategies will be focused on the overarching KPIs and the largest impact area of the business; however, more immediate AI can be targeted differently. For example, brands can use AI-powered tools for customer segmentation, targeting specific groups based on their behavior, preferences, or demographic information. They can implement AI-driven content generation tools to create personalized and engaging copy for email campaigns, social media posts, or blog articles. And brands can adopt AI-based marketing automation platforms to streamline and optimize marketing processes, such as lead nurturing, scheduling social media posts, or sending personalized product recommendations.
Finally, a key role for both short- and long-term strategies is to identify and assess potential AI use cases. While all should align with business objectives, they should also be specific, measurable, and focused on solving business problems or creating new opportunities.
Data Quality and Management
Without data, there is no AI. Whether preparing for an AI-enabled future business platform, or seeking a subset of the business for quick hits, the representative data must be prepared or be able to prep. One caveat to nearly all uses of AI-enabled functions: they depend on large volumes of relevant, accurate data. All else being equal, larger datasets of customer-brand interactions will provide more accurate predictions, recommendations, and ad bidding. Machine Learning (ML) algorithms depend on learning by mining rich, large-volume datasets. For example, toolsets such as GA4’s predictive metrics include thresholds that must be met to be effective. The more data, the more effective and accurate the insights.
Long-Term Data Needs
AI systems require high-quality data to function effectively. Businesses must establish robust data management processes, including data collection, storage, organization, and cleaning. Ensuring data quality and consistency is critical to the success of AI initiatives, as poor data quality can lead to inaccurate insights and poor decision-making.
- Assess existing data: businesses must know the quality and completeness of existing data, along with requirements of data cleaning, integration, and enrichment.
- Build a data infrastructure: ensure data technology supports AI, including data storage, processing, and analytics tools.
Short-Term Data Needs
Short term approaches should either focus on data strength, or on AI practices that do not depend on owned data. For example, public social media data is accessible to sentiment measurement AI systems. Search data is available through SEO AI systems. Chat-bots can learn from data as it comes in. All of these work to help begin an AI journey while preparations are in motion for a robust strategy focused on highest value KPIs, prepped centralized data, and even more specialized staffing.
Technology and Infrastructure
Tech infrastructure for a robust AI strategy requires more than data storage and preparation. It includes the hardware, software, and interconnected systems and communication that comprises an AI platform. For example, a manufacturing company could invest in an AI-powered predictive maintenance system. This system would use sensors and machine learning algorithms to predict when machines are likely to fail and schedule maintenance accordingly. However, to implement such a system, the business would need to have the necessary sensors, data storage, and networking capabilities.
Long-Term Tech Infrastructure
For a long-term AI strategy, businesses must Invest in the right technology stack and infrastructure to support AI initiatives. This includes hardware, software, and cloud resources to store and process large volumes of data. Selecting the right tools and platforms for AI development, such as machine learning frameworks, data visualization tools, and AI platforms, is crucial for seamless integration and scalability.
Short-Term Tech Needs
Implementing complex and varied technologies and platforms is a large-scale endeavor, taking analysis, planning, and expertise. However, existing AI technology solutions allow for targeted implementation of AI capable of producing powerful returns for the bottom line.
The fastest way for businesses to begin using AI to increase performance is to leverage pre-built AI solutions that are readily available in the market. These solutions typically require minimal technical expertise to implement and can be customized to meet the specific needs of the business. Given the overwhelming advantage that toolsets (e.g., machine learning, recommendation engines, chatbots) have over cost, productivity, and accuracy, brands fully utilizing available AI have a competitive advantage over those that do not. Some of the more common AI-enabled toolsets include:
- Marketing platforms: standard platforms include AI-enabled functions to support serving ads, including targeting and bidding. Arcalea’s Galileo attribution solution uses AI to maximize ROI across the marketing mix.
- Predictive Analytics: for brands who’ve moved from Google Analytics 3 (GA3) to Google Analytics 4 (GA4), availability of predictive metrics allows audience configurations that outperform and drive insights beyond manual analysis. Currently, GA4 can calculate predictive metrics for purchase probability, churn probability, and even predicted revenue.
- Chatbots: Chatbots can be used to handle customer inquiries and complaints, reducing the workload on customer service teams and improving customer satisfaction.
- Sentiment analysis: AI can analyze social media and other online content to determine the sentiment of customers towards a product or service. It can be used to monitor brand reputation, identify emerging trends, and improve customer service.
- Personalization: many utilities can help deliver personalized content and recommendations to customers based on their preferences and behavior. Data sources can be immediate (e.g., customer service) or public (e.g., search queries) and drive performance in multiple dimensions, such as customer engagement, brand loyalty, and impact overall revenue through purchasing behavior and LTV.
Talent and Expertise
Long-Term Personnel Skills
Businesses can invest in developing AI expertise within their organization by hiring a skilled team of AI professionals, including data scientists, machine learning engineers, and AI researchers, to develop, implement, and maintain AI solutions. Additionally, brands should focus on up-skilling existing employees and fostering a culture of continuous learning to ensure that the workforce remains up-to-date with the latest AI advancements and best practices.
Short-Term Expertise Needs
In order to leverage AI immediately and in the future, a business must prepare staffing equipped with the necessary skills and expertise to work with AI systems. Not only must personnel operate AI systems, but also learn how to interpret and act on insights generated from these systems. Since short-term goals may be unique to each business staffing environment, brands may select from a wide variety of options and combinations to reach the expertise levels needed.
- Formal specialized AI employee training: providing formal training programs for existing employees may be augmented with new hires in data science.
- AI and Machine Learning Courses: outsourced training workshops may provide background knowledge and applied expertise to existing employees.
- Conferences and Webinars: a broad range of AI-driven marketing trends and strategies are readily available in group and inexpensive settings
Success and the Long-Term AI Strategy
Many of the requirements for an AI-ready business parallel those of a mature data science framework. Data analytics is a requirement for brands in a digital marketplace, whether a large ecommerce or a B2B in which SDRs and their teams drive revenue with offline connections and sales.
Moreover, transitioning from pilot to a scaled solution requires expertise beyond a purchased widget. It requires a framework of knowledge that allows measurement and decisioning to advance beyond one-off implementations.
Piloting and scaling AI projects: Start with small, manageable pilot projects to test the effectiveness and feasibility of AI solutions before committing to large-scale implementation. Once successful pilot projects have been completed, create a clear roadmap for scaling AI initiatives across the organization.
Change management and adoption: Successfully integrating AI into business processes requires a well-planned change management strategy. This involves communicating the benefits of AI to employees, addressing potential resistance, and providing the necessary training and support to facilitate adoption. Ensuring that the organization is agile and receptive to change is essential for long-term AI success.
Measurement and evaluation: Regularly measure and evaluate the performance of AI initiatives against predefined success metrics. This allows businesses to identify areas for improvement, iterate on existing AI models, and quantify the return on investment. Continuous monitoring of AI initiatives helps to ensure they remain aligned with business objectives and deliver ongoing value.
Monitor and measure performance: Finally, it’s important to monitor and measure the performance of AI solutions. This will allow a business to make adjustments and improvements as needed, and ensure that the maximum benefit from the investment in AI.
Finally, even partnering requires a core set of knowledge to ensure that the business internalizes concepts and practices to gain enough autonomy to own strategy and tactics. Partner with AI-focused research institutions, universities, or technology providers to gain access to the latest AI knowledge, resources, and expertise. Join industry-specific AI consortia or networks to share best practices, learn from peers, and stay informed about the latest AI trends and developments. As a brand gains from immediate stock AI implementations, the longer strategic efforts across data, technology, and people will ensure broader impact of AI.