Multi-Touch Attribution (MTA)
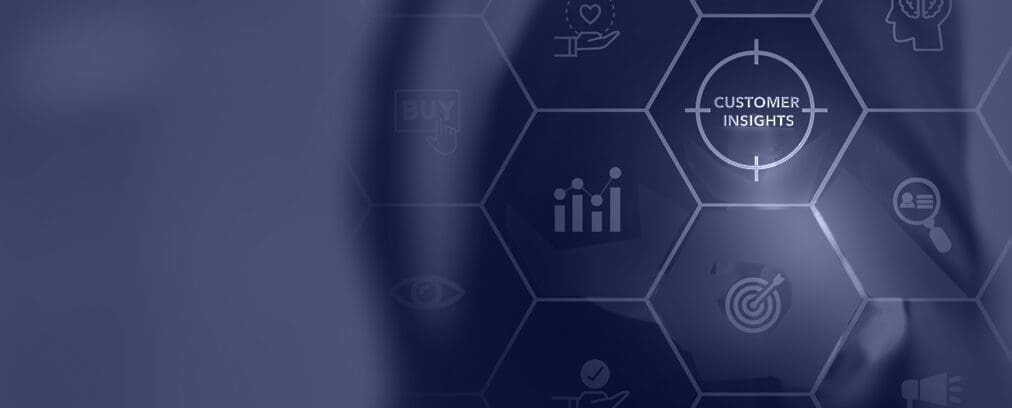
Summary
Businesses strive to calculate the value of marketing elements and gain an accurate understanding of the customer journey. By planning MTA solutions around KPIs, brands can create a critical resource that reveals accurate revenue attribution and specific insights into customer journeys. By connecting true attribution values across dimensions, businesses can query datasets and optimize across countless dimensions and values. While challenges to successful implementation are many, careful planning in design ensures that the output of data-driven MTA is relevant and actionable. By positioning a strong MTA solution within a larger framework, brands can elevate their market position, as well as their digital marketing and data science maturity.
Introduction
The Prerequisite of Measurement
The parallel need to measure marketing value and understand the customer journey have driven brands in search of a consistent, accurate methodology for measuring results from beginning mass-market media. However, the structure of marketing media often drove measurement toward the volume of reach and exposure rather than the specific behavior of customers. As early as the 1930s, when radio advertisers began using exposure-based metrics for measurement, the relative ease of audience exposure measurement drove a standard that has maintained currency for nearly a century.
By the mid-1990s, early digital ad sales were similarly based on exposure, with reach measured in unique pageviews and sold in thousands of impressions (CPM). By the late 1990s, the monetization of search engines drove the adoption of cost-per-click (CPC) as the second digital ad currency.
The Evolution of Measurement Focus
Volume-based measurement can be effective at calculating brand strength, growth, and even opportunity. The 1980s witnessed an expansion of applied large-volume measurement, providing insights beyond traditional reach and exposure. Share of Voice (SOV) began to reveal just where and how market share could be measured and impacted, using the Advertising Intensiveness Curve to identify excess SOV to drive market share. The addressable market could be redefined, and SOV applied to any number of specific metrics to drive revenue through optimized dimensions. SOV is applied to keyword ranking, to SERP ownership, referring domains, in any proxy of marketing reach closer to the customer journey elements.
Similarly, Marketing Mix Modeling (MMM), once the domain of large-scale brand assessment, is now combined with other frameworks to build a full-funnel multi-channel optimized approach. Augmented with different modes of measurement, such as incremental lift and Multi-Touch Attribution (MTA), MMM is now reclaiming a space in organizational toolkits.
The difference in both of these once volume-attuned measurement models is their shift to focus on the detail: the granularity within the customer journey. Either through evolved practices or combining with other models, these re-envisioned legacy approaches gain a more comprehensive view of the customer journey and of marketing performance.
The Rise of Multi-Touch Attribution
Multi-Touch Attribution (MTA) identifies the value of each touchpoint in the customer journey, attributing the revenue fractionally to each touchpoint based on contribution to the conversion. As a result, companies can calculate ROMI at discrete levels, whether at channel, campaign, or specific ad creatives for each journey ending in conversion. And with detailed individual and aggregate customer journeys identified, brands can architect specific journeys to drive results.
Attribution in the Customer Journey
Multiple factors drove the recent surge of interest in MTA, beginning with the nature of digital marketing’s ease of item-level data tracking. While the ad monetization of digital media began with reach and exposure via clicks, digital ad platforms began calculating the value of each touchpoint in the customer journey. Now, increasingly sophisticated ad platforms are mining and using touchpoint fractional influences for automated bidding, more accurate placement, and predictive analytics. Widespread adoption of web analytics platforms, such as Google Analytics, surfaced user-event data and the granular activities within the journey. Eventually, off-the-shelf MTA solutions began to offer companies accessible user-event data for multi-touch modeling and conversion analysis.
Attribution in the Measurement Framework
As a conversion analysis methodology, accurate attribution can achieve two key results: calculate the efficacy of discrete marketing elements within an initiative, and provide a quantitative understanding of the customer journey.
What does it do?
- Estimates the amount of contribution of marketing elements to a conversion
How does it do it?
- Evaluates granular journey data captured by digital tools and analytics
For what result?
- Creates realistic investment optimization and provides a more comprehensive understanding of the customer journey
More recently, MTA has become devalued in some quarters of the marketing ecosphere. Organizations begin with inadequate planning, mismatched software or vendors, or the inevitable challenge of working outside of one’s expertise. As a result, many practitioners land in Gartner’s “Trough of Disillusionment” phase of new solution adoptions. Moreover, before most brands have delved into MTA, large consultancies are already placing the practice within a larger measurement framework, devaluing comprehensive attribution before most have attempted it.
BCG Measurement Framework
Nevertheless, accurate attribution, specifically data-driven multi-touch attribution, provides a fundamental capability to inform and activate strategy. By accurately attributing conversion revenue to customer touchpoints, marketers can isolate and aggregate accurate impact data by any dimension or value. Application of MTA outputs ranges from rebalancing spends between channels to maximizing and accelerating each buyer journey within a brand’s digital footprint.
Still Blocked from Real Sales Attribution?
Accurate lead attribution informs sales across channels and platforms. However, most brands still use inaccurate single-touch models. Get intel on conversion sources, optimize buyer paths, and rebalance spends. Don’t settle for one-size-fits-all solutions.
We’ll build a solution that fits your business.
Attribution Definitions and Models
Marketing attribution terminology and definitions are often used loosely across practitioners, vendors, and consultants. Consequently, a key challenge to applying attribution models is the misunderstanding such ambiguity creates.
The term “Marketing Attribution” is the highest-level attribution and can include all levels of attributing results to marketing, whether at the channel, initiative, or ad level. However, specific attribution approaches are defined from the most basic last-click, channel-level attribution of revenue to data-driven algorithmic multi-touch attribution (MTA).
While the imprecise use of terminology contributes to industry-wide confusion, each type and level of attribution can be easily broken down to create clarity and opportunity.
Attribution Levels
From the simplest attribution, revenue is tied back to the channel most responsible for the sale. For example, using a simple single-touch model, marketers might simply compare the spends and returns from Google Ads compared to Facebook Ads. The cost per acquisition (CPA) between each channel can then drive ad spend allocations.
At the other end of the spectrum, a customer conversion is attributed fractionally to each of the touchpoints that contributed to the sale. The basic channel-level attribution allows marketers to increase spends in high-performing channels and reduce spends in low-performing ones. The more sophisticated multi-touch attribution model can drive specific touchpoint optimizations, adjusting marketing elements down to specific creatives, combinations, sequences, within and across channels, and specific to target segments.
Attribution Models
Single-Touch Attribution Models
Easy to understand and apply, single-touch models are naturally supported by many marketing and ad platforms. As marketers naturally look to proximity to assign attribution, two key transitions are easily captured: when a prospect first enters the lifecycle (first touch), or the touchpoint that finally pushes the prospect to conversion (last touch).
Single-touch models credit 100 percent of the conversion to one marketing touchpoint, with all other touchpoint influences disregarded. In an inexpensive e-commerce commodity offering, a single touch may capture the critical touchpoint. However, in a longer B2B cycle, the middle touchpoint in which customers review product specifications may be undermined as a key driver of conversions.
Ultimately, single-touch attribution models do not provide insights into how different touchpoints across the funnel interact and contribute to moving prospects to conversion. While 20 years ago the average number of touchpoints was two, now it’s easily 20 or more (Google).
First-Touch: the first touch or interaction that drives a user to your website can include anything from an organic search to a Facebook ad. In each case, the first touch receives the entire attribution of the conversion, ignoring all touches between it and the conversion. As the initial awareness of the customer, first touch focuses on driving initial traffic. Easy to capture, first touch can be found in traffic sources or referrer pages in Google Analytics. However, it fails to capture repeat visits throughout the remaining customer journey.
Last-Touch: the last touch or interaction before the conversion focuses on the bottom-funnel high-return touches. However, it fails to capture any earlier influences leading to the last touch. Similar in complexity to first-touch, last touch is also easy to configure, as the source or touch preceding the conversion is easy to capture. Last-touch is easily found in Google Analytics or typical e-commerce platforms. While failing to account for journey complexity, last touch allows marketers insights possible from comparing last touchpoints of different conversions.
Multi-Touch Attribution Models
Unlike single-touch models, multi-touch attribution models evaluate the impact of each touchpoint leading up to a conversion. By calculating the fractional value of each contributing component, MTA can provide insights into marketing efficacy, customer behavior, and optimization opportunities against a wide range of contexts. The resulting application can drive ROMI through micro-optimization of customer journeys to drive revenue across different paths, channels, and even target segments.
Linear: The simplest multi-touch model, linear attribution assigns equal credit to all touchpoints before conversion. While eliminating arbitrary differing allocations across touchpoints, the equal allocation is itself arbitrary, as the model is unlikely to accurately gauge the real influence differences across touchpoints. For example, in a model where initial acquisition is the most critical step, the first touchpoint will not receive appropriate weight.
A linear model can help marketers that are learning the customer journeys undertaken by customers, providing a baseline understanding and revealing the different channels used. With enough volume, brands can identify those channels that often recur, and those that are outliers for increased or decreased performance.
Time-Decay: The time-decay model applies more weight to touchpoints closest to the time of conversion, discounting the influence of early interactions. Emphasizing conversion-adjacent touchpoints is more closely aligned with long sales cycles but limits marketing touchpoints at the top of the funnel when customers are first attracted. The time-decay model is more applicable to long B2B relationship-building sales cycles in which later touchpoints have more importance, but less helpful for shorter B2C journeys.
The time-decay model highlights the touchpoints driving conversions, as well as the channels that lead to the penultimate touches. While it reflects the final elements of the journey, it fails to capture the awareness-driving channels and early differentiation messaging.
U-shaped: This position-based model credits first and last touchpoints heavily (40% each), with middle touchpoints sharing the remainder of credit. This model focuses on the top and bottom of the funnel (initial acquisition and conversion touchpoints), with less focus on engagement activities in the middle of the journey. As it covers two of the critical phases–initial visit and conversion–this position-based model provides a more inclusive combined first/last touch model.
The U-shaped model identifies key traffic acquisition and conversion-channel touchpoints, but limits focus and value on most nurturing interactions that could reconnect the brand and customer.
W-shaped: Similar to the U-shaped position-based model, the W-shaped model focuses on initial acquisition, the conversion touchpoint, and the midpoint engagement (e.g., lead conversion) with equal 30% credit, with remaining touchpoints dividing 10%. The W-shaped model focuses on three key stages in the journey–acquisition, engagement, conversion, or initial visit, lead, sale.
Again, based on a brand’s sales cycle, the W-shaped model is effective when the key initial visit, the lead conversion, and the opportunity close are clearly established. The W-shaped model focuses on touchpoints that generate interested traffic, create a lead, and drive the final conversion, and works well with matched B2B cycles.
Full-Path: The full path model provides a more detailed full-journey approach, reviewing initial touchpoints and all other touchpoints up to the conversion. Unlike the W-shaped position model that captures 3 key stages, the full-path model goes one step further, capturing all elements of first touch, lead conversion, opportunity creation, customer close, with 22.5% to each of the 4 critical touch points. The remaining 10% is distributed across touchpoints between each of the key phase points. However, full-path capture, once in place, allows marketers to change the weights of each touchpoint within the journey. This is, of course, custom attribution modeling, and one reason why full-path modeling is sometimes confused with custom.
Model Application
Applying Rules-Based Attribution Models
Rules-based models are not completely arbitrary; in fact, experienced digital marketers may base their model choices on a combination of data-driven and subjective experience. The attribution choices may be driven by knowledge of specific campaigns with a long historical record. For example, feedback from the B2B sales team and from CRM records could reveal that prospects who schedule a consultation become customers 40% of the time. Or that leads who download the white paper comparing all competitor options leads to a consultation 25% of the time. In each case, those touchpoints (last and mid-points) would be weighted to align with historical data.
While multi-touch models more closely approximate customer journey elements than do single-touch models, even multi-touch rules-based models are usually based on arbitrarily applying the models to flows that may divulge in ways not apparent to the marketer or sales team. A successful, accurate application of attribution models should reflect the data revealed in the business context. While many inexpensive MTA software packages capture journey data and apply models to customer journeys, when models are applied arbitrarily they fail to produce actionable outputs.
Applying Custom, Data-Driven, and Algorithmic Models
While linear and position-based models are more likely to align with rigid rules of conversion attribution, custom, data-driven, or even algorithmic models are designed to more realistically reflect a business reality. In fact, a likely first step to data-driven modeling is an existing rules-based model modified using specific brand data.
Ideally, all businesses should use some degree of data-driven attribution. Subjective marketer experience is no match for machine-learning algorithms processing complete sets of marketing data for customer journeys.
Custom: A custom model provides exacting control over how different touchpoints receive conversion credit. Organizations with distinct marketing and sales activities across more consistent and defined cycles may use these insights to develop a custom model that aligns more closely than the above rules-based models. However, this requires a significant understanding of a brand’s channel activity and data. With an approach informed by data and experience, teams may design touchpoint allocation models that more consistently map to the efficacy of the actual cycle. More sophisticated full-path custom attribution models may include view-throughs, whenever ads were viewed and not clicked. However, unless the model is continuously tested and realigned, even custom attribution will not account for customer idiosyncrasies, counter-intuitive paths, and ongoing change within the brand’s market.
Weighted Multi-Touch Model: A weighted MTA model accounts for all touchpoints within the customer journey, allocating specific weight to each touchpoint using consistent data-driven metrics. For example, a model may determine that the duration of a touch, such as the time on page for a brand’s website, could be represented by a specific multiplier. Similarly, touchpoints that fall in a specific sequence, such as after a paid Search Ad could be another weighting factor based on historical data correlated with conversion probability. This type of data-driven model provides an accurate view of how each part of the journey is working; however, the effort required to design and apply the model is challenging.
Algorithmic: Data-driven MTA models leverage individual-level user-event data across a company’s buyer experience of all touchpoints to calculate the amount of credit for a specific conversion attributed to specific marketing touchpoint influences. Machine-learning attribution models outperform rules-based, and with continued relevant data volume, get better at allocating credit and identifying insights beyond an analyst’s ability. Models like Shapley Value can determine the contribution of each touchpoint within a journey. Markov Chain can determine the probability distribution of any next state based just on the current state using a transition matrix.
In this example from Google, the Shapley value determines the value of a Display touchpoint through the removal effect. As of 2023, the default model in Google Analytics is data-driven attribution, or algorithmic.
Applying Models Contextually
Often the standard attribution models are presented as a rigid rules-based structure in which touchpoints become “key” based on positioning rules rather than the context of brands and their specific sets of customer journeys. In such a structure, the W position-based model would recognize first, last, and midpoint as the key touchpoints for attribution and analysis. However, accurate output depends on recognizing (from both individual and aggregate) a reality of business context. The goal is to gain accurate attribution in order to perform more complex queries to uncover multiple optimization paths. Such optimization increases the odds of specific responses or returns. The attribution in itself means little if the output of the model can not be extrapolated against key dimensions impacting KPIs.
An intentional and informed application of a somewhat static model can be effective, but a default application would devalue important touchpoints and elevate non-critical ones. The goal in MTA is to accurately identify key touchpoints that play significant roles in the customer acquisition process, for example, those touchpoints that signal a phase change within the customer journey.
Marketing Impact Demands Accurate Data.
Accurate sales attribution results from strong user-event data. However, many companies do not capture data identifying key touchpoints. Use our MultiTouch Attribution to track all touchpoints, accelerate journeys, and boost sales.
We’ll maximize your competitive advantage.
Drivers for MTA Adoption
Quantifying marketing impact and understanding the customer journey are prerequisites for marketing decisions. Without such data, brands are using a shotgun approach to marketing spends, resource allocation, and sharing data with customer-facing teams. However, additional obstacles to achieving clear, closed-loop marketing and comprehensive views of the customer drive the critical need for MTA solutions.
Accurately quantifying what works, to what degree, and in what context is a fundamental part of a business’s strategy and tactics. The insights derived from a full accounting of the customer journey–at the individual level and aggregate–drive a deeper understanding of the customer, the needs, behavior, and methods to meet them.
Complex Journeys
While marketers have visualized the journey as a linear flow, left to right or top to bottom, the buyer journey encounters countless detours in different directions. An explosion of channels, platforms, devices, and user-generated content has customers interacting in far greater volume and variety. What was once conceivable as a simplified linear flow is now often represented by scattered touchpoints across a hurricane of pathing options.
Marketers and analysts have long known that customer behavior can be irrational, emotional, and counter-intuitive. Yet paired with siloed measurement approaches, the increasingly complex and evolving journey highlights the limitations even further.
A complex marketing mix requires better measurement than siloed metrics.
Complex Marketing Mix
To meet the complexity of the customer journey, companies invest significantly in numerous paid and organic marketing approaches, all requiring significant resources and needing discrete and integrated ROMI to measure efficacy and to optimize tactics. Numerous ad networks, content marketing, and SEO all have significant resource commitments. And each demands allocation decisions based on far more data than quarterly channel-level revenue against monthly ad spends. In order to accurately attribute revenue, specific data variables like ads clicked or blogs read must be mapped to initiative spends accurately. Without comprehensive consumer touchpoint data informing conversion analytics, complex marketing mixes become a large aggregate spend and return without decisioning information.
The original Marketing Technology Landscape project to document the field began in 2011 with 150 marketing technology solutions. In 2022, the list includes nearly 10,000 applications in 49 categories.
Legacy Measurement
Established or legacy measurement practices are often not designed for the complexity and fluidity of consumer journeys today. Digital marketing began focused on desktop users, their site visits, page views, and bounce rates. Later digital analytics exposed other visitor actions within a flow across a company’s website. However, these detailed flows were often in walled gardens, or tied to channels, platforms, devices, and vendors. Mobile apps transformed these analytics again, bringing new variances in measurement approaches.
Much of legacy measurement is built around single channels, in organizational data silos, using dated processes, and even legacy systems that reinforce the compartmentalization of measurement. As a result, widely used assessment methods fail to meet key goals:
- To apply consistent measurement principles across widely varied contexts
- To achieve a singular source of true attribution to drive all types of measurement
Inaccuracies driven by legacy measurement drive up the cost of acquisition, lagging behind advances in reach and growing addressable markets.
Offline/Non-Click Modalities
Extended B2B sales cycles often depend on offline touchpoints. Increasingly, offline, podcasts, and other non-click modalities are blended into marketing campaigns. Brands that have powerful offline touches require a model and system that captures and aligns journeys that may flow in counter-intuitive ways. MTA approaches are now using multiple mechanisms to capture touchpoints for phone calls, meetings, conferences, as well as the incremental lift from podcasts, radio, and television broadcasts.
Privacy Restrictions
Privacy-centric regulations and expectations are already undercutting third-party cookies and mobile ad identifiers, core components of many measurement models. While numerous reasons exist to transition from the current confused and misaligned traditional approaches, organizations are slow to move away from even a complex, underperforming current state. However, the increasing privacy-focused environment upends existing practices.
New solutions and tracking workarounds are often modality- and vendor-specific. And each company’s unique digital footprint and marketing practices may make off-the-shelf measurement solutions hit or miss. Conversely, many MTA solutions are based on first-party data (the conversion) that is then tied back to matching anonymous identifiers from prior touchpoints.
Spend Justification
While often the first driver listed on MTA software landing pages, justification of spends is likely the least critical driver for investing in an MTA solution. Marketing cost centers often play defense. However, element-level ROMI not only accurately allocates department-level spends, but it also creates the insights needed for marketing optimization.
Together, these adoption drivers confronting current marketing departments and their brands create barriers to continue existing measurement approaches. Consequently, these challenges push brands to extend their impact with accurate true attribution–the ability to identify and quantify which marketing elements are creating value while surfacing a detailed understanding of the customer journey.
Benefits of MTA
While aforementioned drivers threaten a company’s current state practices, MTA increases capabilities that elevate brands with new advantages spanning the organization.
Measure Beyond ROAS and Simple ROMI
Whether ROAS, ROMI, LTV, or CPL, if revenue is not accurately attributed to specific marketing spends, the most powerful metrics are approximates for internal comparisons–not performance. Return on Ad Spend (ROAS) does not typically account for attribution. Rather, it is a simple comparison between spends and revenue.
ROAS = Revenue/Spend
As a result, ROAS cannot tell marketers anything about marketing impact. Just what they already know in a convenient metric. Brand spends $10k in monthly advertising, and receives $20k in monthly revenue. The ROAS is 2.
Return on Marketing Investment (ROMI), however, requires attribution. And the more detailed ROMI desired, the more necessary a full MTA model. With more complete attribution data, ROMI can fully account for spend, narrowing the return accuracy. In short, ROMI quantifies the amount of return beyond the cost.
Typically ROMI is the financial value generated by a marketing initiative or set of initiatives, represented by a simple formula:
Quantifying channel-level ROMI is far easier than the attribution required to calculate ROMI for cross-channel marketing or customer journeys that encounter multiple touchpoints falling under different channels, initiatives, or even types of investment (e.g., SEO, SEM, content marketing, etc.). Yet, the vast majority of customer journeys do, in fact, include touchpoints beyond one initiative, channel, or type of investment. So which ones should be included in the “Cost of Marketing” variable in the ROMI equation? Determining which costs are included (calculating the “Cost of Marketing” variable) requires accurate multi-touch attribution.
The same is true for accurate lifetime value of a customer (LTV), the customer acquisition cost (CAC), or any other marketing return metric that relies on accurate inclusion of discrete marketing element costs and the amount each element influenced the eventual sale.
True attribution zeroes in on the marketing activities responsible for specific resulting actions, whether an online purchase or scheduling a consultation that leads to a sale.
Allocate Costs Accurately
Multi-touch attribution reveals what works across the organization’s marketing and sales touches, from department-level spends, to initiative, and actual creatives. Attribution specificity allows accurate allocation of costs between initiatives, between sales and marketing, and actual ad creatives and messaging. By learning what works across the org, the entire business is accountable.
Connecting touchpoints to revenue contribution allows accurate allocation of spends based on return. By defining cross-functional KPIs, the resulting quantification and recommendations are shared. When marketing and sales access the output of MTA, collaborative efforts are aligned.
Close the Marketing Loop
A minimum requirement for the accurate calculation of ROMI, closed-loop marketing requires capturing and accurately connecting each lead, customer, and revenue transaction to the marketing components that enabled them. In the past, a large measure of the work was ensuring that marketing was aligned and connected with sales, and that these data elements were captured together.
With MTA, these elements are captured at a granular level that provides more alignment than just the correct collection of items. MTA can quantify each data element (including initial entry into top-of-funnel, the conversion to a lead, the conversion to an opportunity, and the conversion to a customer). When the CRM record captures touchpoints, and the MTA solution calculates the fractional value of each element to the ultimate sale, the sales team has actionable data. And that data aligns with different customer segments and sources–along with accurate ROI identifying which leads are likely most valuable. MTA provides a granular level of closed-loop marketing that is quantifiable and actionable.
Complete 360 View of the Customer
Understanding your customer goes far beyond learning that a certain demographic responds well to frequent relevant social media updates. The gold standard of customer relationships, the 360 view of a customer, combines all customer information including purchase and brand interaction history, demographics, geographic, and additional behavioral, all in a centralized source accessible to all customer-facing employees. However, combining a full-path MTA model with existing record data creates a powerful granular level of insights. When captured and accessible to key staff (marketing, sales, support), more brand interactions will be worthwhile to consumers and profitable for the company.
Understand the Customer Journey
Opportunities from an aligned MTA measurement can optimize specific customer paths and messages for different segments and buyer phases. With an increasingly complex customer journey, marketers need an MTA solution to capture and quantify the impact of each touchpoint as a prospect becomes a customer. Measuring individual and aggregate journeys reveals patterns that drive insights and optimization. Identifying how each touchpoint fits into larger buyer discovery, marketers can use trends to reveal efficiencies and obstacles. Full-path MTA can uncover elements that move a visitor to become a lead, to transition to an opportunity, and become a purchasing customer. When queried in alignment with cross-functional KPIs, this quantified journey can be applied to decrease CAC, refine messaging, and even optimize offerings.
Increase Revenue
With attribution, marketers expect to rebalance spends across channels, increasing effective spends and decreasing less effective spends. By calculating intersections of journey attributes and resulting transaction values, marketers can augment and refine across dimensions to drive revenue. Intelligence extracted from customer journey data can speed time to conversion, reduce CAC, and produce revenue faster.
Calculating the costs of touchpoints and their conversion values, marketers can query multiple dimensions against the dataset to isolate revenue drivers.
Increase Effectiveness
When the full journey and its elements can be explained, brands can identify and redeploy the key touchpoint sequences that drive revenue. Using techniques such as Markov Chain to determine touchpoint probability and Shapley value to determine the contribution of each node in the journey, analysts can provide marketers with the information necessary to architect measurably better-performing customer journeys.
Moving a white paper that drives opportunity conversion above the fold, marketers can transfer quantified performance to better reach leads when they need it. Once a replicated journey is understood at the individual and aggregate level, targeted queries can isolate and repurpose key marketing elements for specific performance improvements:
Conversion analysis: Intelligence on conversion sources
Anomalies: Detect anomalies and streamline paths to conversion.
Rebalance: Reject low-performing path configurations and standardize powerful optimizations.
Finally, by running queries related to KPIs (the foundation of your MTA design), marketers uncover unexpected insights. Drive new tactics to prospects and teams: deliver unlocked insights as context-specific variable sets to prospects.
Rather than overall ad spend and return by channel, touchpoint level analysis reveals how efficiently each component within channels moved prospects toward conversion. MTA can identify which components were most effective in acquiring visitors at top-of-funnel that eventually converted; which channel touchpoints were most effective in mid-funnel, converting lead to a successful opportunity for sales; and even what elements sales uses most effectively to complete the purchase.
Still Using Last-Click Attribution?
Today’s complex marketing mixes expand reach across channels and platforms. But without accurate attribution data, the relative value of each touchpoint is unknown. Use our MultiTouch Attribution to increase ROI 20-40%. Let us maximize your spends.
We can expand your capabilities.
Challenges to MTA Success
Multi-touch attribution can provide fundamental decisioning information across organization functions and customer journeys. Moreover, the broad utility of MTA can be a source of actionable data for any brand. Nevertheless, many organizations either do not attempt adoption or implement a solution misaligned with the business reality.
User Event Data
Multi-touch attribution models depend on event-based data tied to specific individuals. Once a user is identified at conversion (purchase info, contact forms, etc.), the software must match the identified user with the prior anonymous ID user events. This connection allows marketers to close the loop. This is a key obstacle to implementing MTA or gaining attribution-enabling data: most companies do not track different touch points connected to an individual.
Rules-Based Defaults
Off-the-shelf attribution tools usually come with default settings that become familiar quickly. As a result, practitioners may be reluctant to experiment with additional, more complex (and applicable) models that more closely match the business need.
Rules-based models are easy to understand and explain to stakeholders, and may be very mappable to a business model or a specific initiative. For example, a U-shaped model is accessible to anyone. The prospect arrival and conversion from lead to customer capture two key phase changes. Explaining machine-learning algorithms which may require time for learning and testing cycles, is far more difficult.
Flexibility Requirements
A successful MTA implementation must be flexible and quickly applied to live initiatives. To gain traction, the model and execution must match specific needs of a business’s varied customer journeys, work with all the existing marketing elements, and apply insights in real time. If stakeholders cannot see how a solution applies to their world, the necessary cross-functional support will never fully materialize. And rightly so, while multi-touch algorithms can create tremendous gains, without models that match needs, accurately quantifying discrete marketing elements is unlikely.
Instead, multiple models with on-the-fly adjustments should allow customers to “what if” the customer journey in order to tune marketing and sales activities.
If marketers aren’t running multiple models and viewing campaigns from several perspectives—models and algorithms—they are unlikely to find which are better at different parts of the funnel, or for different segments. All depends on matching the opportunity of specific marketing mix complexities.
Realtime optimization is quickly becoming a requirement. Successful practitioners experiment with advanced models, and continuously recalibrate their approach.
Inadequate Planning
One reason for the increased application of MTA is the ease of capturing data within digital marketing platforms. However, the ability to track data elements often exceeds any plan or ability to apply the data to solve marketing problems. Defining goals, the queries needing answers, and the KPIs on which to focus is mandatory for a useful MTA solution.
Planning an MTA Solution
MTA success depends less on the complexity and number of components and more on planning that targets the specific KPIs needing analysis. Nevertheless, brand readiness depends on digital analytics experience and partnerships. Implementing a custom solution internally relies on personnel and infrastructure levels, data science maturity, as well as a willingness to test and experiment.
Organizations without a CRM or a dedicated analyst will likely have challenges internally developing an MTA solution. Brands that are “winging” business analytics, or are entirely dependent on an insight-generating BI visualization tool, will probably need to outsource this.
MTA Structure and Process
MTA Components
The core of an MTA solution can be conceptualized as three key components:
Data: all the variables in the customer journey, marketing and sales touchpoints, spends, revenue
Models: all applicable models, e.g., first, last, W, U, full path, data-driven, algorithmic ML-driven
Measurement: all the identified KPIs you need to calculate to achieve your attribution goals, articulated in the form of specific discrete questions against data views
MTA Solution Structure
Process
However, to make the MTA solution work, a goal-centric planning phase and a post-measurement application phase ensure that the design is accurate to the business practice from data capture to action. Front-end planning ensures applicability on the back end. The KPI definition drives success across the model. Without clear KPI definition, the data elements, the applied models, and the data transformation capability for measurement will likely not match needs or expectations.
PLANNING
Define KPIs, what you want to find out.
DATA
KPIs determine the data elements to capture, and how they must be connected.
MODELS
Define MTA models to apply to the data set
MEASUREMENT
After all elements are combined as a single source, with basic transformative capabilities, core measurement questions are answered
APPLICATION
From the core answers, immediately actionable insights are applied to marketing elements
MTA Solution Process
The goal-centric planning phase defines the data element combinations that must be captured to enable model application and measurement. The actual scale of a system may vary depending on resource capacity and investment; however, the goals should be clear in the form of KPIs relevant to all brand goals.
Defining KPIs
While digital marketing is often focused on the discrete clicks, localized KPIs closest to the sale or conversion, planning should consider a broader scope than bottom funnel. Planning should define the organization’s KPIs across sales phases, platforms, initiatives, and even functions. To answer questions with actionable information, marketers must determine the dimensions for each KPI.
Example Digital Marketing KPIs and Dimensions
KPIs
- Conversion rate
- Click-through rate
- Sales cycle length
- Transaction value
- LTV
- CAC
- ROMI
- Keyword Ranking
Dimensions
- Cross-referenced KPIs
- Channels
- Initiatives
- Branded/Contextual
- Messages
- Sales Phases
By accurately allocating fractional credit for conversions back to marketing touchpoints, KPIs can be quantified across a wide range of planned dimensions, answering specific questions about KPIs across channels, phases, and the organization.
Example Queries
When data design is founded on KPI elements, relevant queries provide optimization opportunities across dimensions directly impacting revenue and goals.
ROMI by dimensions: If an ultimate goal is to increase ROMI, brands can quantify distinct component ROMI. The result provides optimization data for specific marketing elements:
- Segment(s)
- Specific path combinations
- Compared to CAC (e.g., are instances of high CAC rewarded by substantially larger transaction values or LTV?)
Sales Cycle by dimensions: If an ultimate goal is to speed sales cycles, brands can quantify journey duration against dimensions:
- Overall, shortest, longest by multiple dimensions
- Highest transaction value within short durations
- Highest transaction value within long durations
- Sequencing and combination optimizations by measuring average durations from first visit to lead conversion, from lead conversion to opportunity creation, and from opportunity creation to conversion to customer
CAC by dimensions: If an ultimate goal is to reduce customer acquisition cost (CAC), brands can quantify CAC by all key controlled dimensions:
- Lowest cost touchpoints with median or better conversion rates
- Lowest cost touchpoints with the lowest cycle time
Funnel Phase KPIs
Consider the phase-specific KPIs that would be actionable when quantified: Which marketing elements drive overall organic search traffic, converting organic search traffic? What is the CTR on display ads by campaign or creatives? What are the highest-performing lead converting touchpoints for top-of-funnel interactions? Which mid-funnel touchpoints convert to opportunity? Are specific webinar views, white papers downloaded driving disproportionate final conversions? Finally, bottom funnel demo scheduling, in-depth product guides, and quote requests should be viewed within highest performing sequences.
Offline Touchpoints
If offline touchpoints are a significant driver of consumer phase change, they should be included in KPIs. New approaches connect traditional marketing initiatives and components to online and offline outcomes, whether television, print ads, or phone calls. Many solutions automate the capture of phone contacts, and offline ads can include a short customized link that identifies the source that drove the click.
KPIs across Functions
Ideally, KPIs should include metrics that represent the complexity of spend and return across the organization. If performance depends on functions beyond marketing, their inclusion in KPI definition and the MTA solution is necessary for success.
A naturally aligned function, Sales, is often not included directly throughout the definition of the KPIs or the solution. If a business is segmented heavily between sales and marketing (e.g., sales development reps, business development reps), any attribution model would need to include them. Without buy-in to the model, marketers are speaking for a significant contributor to the revenue without their participation. Capturing the phone calls and touchpoints sales drives is a fundamental part of performance measurement and optimization.
If planning fails to capture a holistic view of KPI contribution across the organization, successes and failures will not be viewed jointly. Even worse, no consensus will exist for where to improve or abandon different customer cycle activities. If measurement is used only to justify the marketing spends silo, then accountability is limited. If functions-wide attribution was accurately enabled, all of the organization could be accountable, responsive, and performance-focused.
Building and Applying MTA
Once KPIs and required dimensions are defined, data must be captured and connected, stored in a database allowing transformations by dimensions and values. The resulting data store is responsive to queries that quantify specific combinations of attributed touchpoints and the KPIs they define.
Execution can always be challenging. Using tools and processes in new ways brings unexpected obstacles and modifications mid-build. However, if built around the identified KPIs and relevant dimensions, the output will be relevant and actionable.
The key to a flexible, KPI-inclusive attribution solution is event-user data, captured in a way that allows connectivity across different dimensions as defined in the planning phase. The starting point is event user data, revenue actuals, and spend actuals, all connected in order to perform queries or even dashboard data transformations by different dimensions and values.
Data Capture
Methodologies for data capture, storage, and transformation can vary but should be based on industry standards that are at low risk of interruption and include redundancies for data-matching capabilities. For example, first-party data is a core of many systems, and is not at risk due to privacy safeguards. Other standard technology in MTA solutions includes JavaScript, UTMs, APIs, public and custom machine-learning algorithms, cloud warehousing, and technology with broad user bases.
To measure the defined KPIs, key data points must be captured and connected in order to be measurable and actionable.
Example Data Capture
CORE DATA
Event-user data: All user interactions with the brand during the customer journey, including user-events in each channel, ad platform, business websites, offline
Connect conversions to revenue: All conversions connected to revenue actuals
Connect individuals to conversions: While e-commerce automatically connects customer identifiers to conversion, B2B in-person conversions might require manual entry into CRM.
Connect converting individual to prior touchpoints: Connect converter identifier with prior anonymous identifiers for touchpoints captured before customer identification at lead conversion; algorithmic data matching can connect prior unique identifiers with a converted individual’s data.
Connect spends to conversions, connect spends to touchpoints: The level of connected granularity enables the measurement of different KPIs. For example, spends tied to channels provide insights into channel-level efficiencies, while spends tied to touchpoints can quantify more accurate estimates of specific path costs and CAC. Several methods are used for spend allocation, including 1) aggregating paid platform spend data across each recorded touchpoint, 2) weighting objective measurements such as time on page, percent time of each step, etc., 3) combinations of true costs where data exists, and weighted costs for other touchpoints.
Dimensions and Values: A core data store and management toolset would include all applicable dimensions and values necessary for detailed queries about a company’s KPIs. Most of the dimensions exist as metadata captured simultaneously with user-event data, and include event time (to calculate time per touchpoint, phase, journey, and any intra-journey durations), channel detail including campaign, ad set/ad creative, keywords, etc.
Data Transformation
The ability to manipulate data views produces the measurement value. Relevance for specific KPIs is explored by combining, sorting, and comparing aggregate and individual record data by dimensions (specific touchpoints, sources, transaction types, product types, segment definition, etc.) and values (# of touchpoints, transaction value, journey duration, etc.). Because the data capture is based on a company’s KPIs, the sorting and filtering of data by dimensions and values answers key performance questions about the effectiveness of past, current, and future marketing elements. A key for data manipulation is the ability to ask “what if” questions, such as, “What if the number of visitors using the shortest journey path were increased by 20%?”
A data store system should be able to:
- Transform/configure data views using specific attribution models
- Configure data views by dimensions and values
- Aggregate and isolate by various dimensions and values
Accessibility and Ease-of-Use
Attribution platform value is driven not only by the configurability of data dimensions and attribution models but through ease-of-use, such as the ability to template specific analyses, and the ability to share data snapshots and different reporting outputs (path analysis, funnel analysis, cohorts, etc.).
Model and Measure
Model application should be flexible, and “on-the-fly,” able to be applied to a data set to explore how customer journey models vary based on the filtering lens. For example, the use of multiple MTA models at once in different campaigns can reveal which best represents different parts of the funnel, with each phase’s discrete goal.
Conversion Journey Analysis
Once data is accessible with configurable views, marketers and analysts can apply queries to answer KPI-related questions to identify specific unique actionable data insights. Once MTA models are applied, measurement of KPI components can be divined to isolate specific touch points, cycle times, path barriers, and comparative efficiencies between conversion journey elements.
- Calculate ROMI at channel, initiative, and creative levels.
- Calculate shortest consistent paths to conversion, comparing by segments, sources, and other major dimensions.
- Identify messages and mediums most often found between visitor stage to lead stage, and lead stage to conversion.
- Identify content types (message keywords, content object types) that reveal high correlation with conversions, or high correlation with above-median transaction values
- Template or automate analyses that repeat previous analysis tasks (isolating types of outliers, grouping sets of dimensions with values above median, etc.).
Apply Learnings from Analysis
Once accurate data is accessible for key questions driven by brand KPIs, marketers can apply the comprehensive understanding of the marketing efforts to improve performance in real time. Because the planning and data capture steps are led by agreed-upon KPIs, the quantitative questions lead to actionable insights.
- Increase ROMI by rebalancing spends across channels.
- Reallocate spend priorities based on marketing components and sequences revealing the strongest LTV.
- Empower sales and marketing with messages and mediums with higher success rates for moving prospects toward conversion for specific segment types.
- Boost spends on content types with higher correlations to conversion, or higher correlations to maximum transaction values.
- Remove or modify obstacles that appear as last touch for a significant volume of non-converters.
- Architect tactics from across top buyer journeys to drive the greatest return across target segments.
If a fully-implemented CRM is part of the MTA data store, the behavioral data from specific journeys can be combined with geographic, demographic, and psychographic data aligning with the value proposition. The ability to continuously update and expand target personas refines targeting with each new wave of data and insights.
The scalability of attribution allows brands to access levels of analysis matching either their organizational data science and digital marketing maturity or matching organizational readiness and willingness to invest. Manage the data starting with the data you can manage, even if the most basic and essential (the final touch). Start somewhere and build out and grow capability.
Positioning for Growth
Gaining a full window into the accurate attribution of conversions to marketing elements can be a gamechanger for companies. However, as recent studies (BCG, McKinsey) have pointed out, MTA is most impactful within a spectrum of measurement tools and practices–what Forrester calls “Unified Measurement” and what Gartner termed “Total Marketing Measurement.”
Organizations can maximize impact by applying all key elements of measurement together, strengthening brand performance within the journey, across the funnel, and within the overall market positioning.
Start where Capability Exists
Multi-touch attribution is demanding, requiring a detailed spectrum of KPI definition, the capture of exhaustive data points of connection, and the configuring of data using critical dimensions and values. Marketing departments must begin where they are organizationally–not necessarily from a technical capability, for that can be offloaded. However, brands should ensure their data science maturity is capable of acting on the data.
Validate Goal, Strategy, and Tactics
Businesses should focus on the clear definition of business goals and strategy before overcommitting to specific measurement methodologies. Validate brand goals, strategy, and tactics for accuracy and alignment first. If analytics from any specific area call these fundamental definitions into question, companies can revisit fundamentals, offering attributes, and funnel back into product dev teams. If a full analysis of goals, strategy, and tactics has not been performed in the recent year, a GST assessment is likely overdue.
Measure Market Position
A brand’s position in the competitive market is reflected through hundreds of dimensions. The Quantitative Market Assessment (QMA) is the methodology to assess every measurable dimension, including competitive market approaches, information architecture, Share of Voice (SOV), technology stack fingerprinting, keyword ranking analysis, and machine-learning analysis of search ranking factors, as well as other brand-specific dimensions. Using a brand-specific competitive assessment, a business establishes relative competitive context across key market dimensions.
Brands that measure their quantitative place on the continuum of key competitive dimensions know exactly where to apply resources to succeed, how to measure results within specific dimensions, and where to adjust to improve performance.
Test and Experiment
A culture of test and experiment surfaces opportunities that cannot be found through traditional measurement. Regardless of business and current measurement approaches, MTA should follow a test/learn approach with different models. Businesses should verify how models perform against specific metrics: efficiency for performance, coverage across segments, across devices, across platforms. Your MTA model may need to be complex to drive the best performance across a complex marketing organization and deployment.
Invest in Personnel
In addition to dedicated analyst positions, marketing should invest in higher capabilities, with measurement roles reporting to C-level/CMO. Visible changes at the top signify a culture shift in measurement importance and the importance of data science. Marketing teams should be more financial and data science knowledgeable. Lack of interpretive skills and framework knowledge limits marketing teams to software-only approaches and a dependency on agencies.
Find Responsive Partners
Marketers need agency partners who excel in marketing data analysis, partners who assist brands in defining data needs, capturing and transforming data at the right granular levels, and applying it to tests and large-scale implementations. For example, Arcalea’s Galileo attribution may be the advantage you need to begin full-funnel omnichannel MTA. As companies partner with transparent and flexible experts, the internal capabilities they build will bring brand strength to new minimum performance expectations.